If you have questions, check out our Github or get in touch via api fxcm. Third Party Links: Links to third-party sites are provided for your convenience and for informational purposes only. FXCM bears no liability for the accuracy, content, or any other matter related to the external site or for that of subsequent links, and accepts no liability whatsoever for any loss or damage arising from the use of this or any other content. Such sites are not within our control and may not follow the same privacy, security, or accessibility standards as ours. Please read the linked websites' terms and conditions.
Risk Warning: Our service includes products that are traded on margin and carry a risk of losses in excess of your deposited funds. The products may not be suitable for all investors. When we have no position at all, this is referred to as being flat. Long positions make money when market prices are higher than the price of the position, and lose money when market prices are lower than the price of the position. Short positions, conversely, make money when market prices go down from the price of the position and lose money when market prices go up from the price of the position, hence, the well-known ideas of buy low, sell high, and buy high, sell higher, and so on.
Algorithmic trading in less than lines of Python code – O’Reilly
Multiple buy executions, or multiple sell executions for different amounts and prices, cause the overall position price to be the volume weighted average of the execution prices and quantities. Open positions are marked to market to get a sense of what the unrealized Profit and Loss PnL of the position is. This means that current market prices are compared to the price of the position; a long position where market prices have gone up is considered unrealized profit, and the opposite is considered unrealized loss. Similar terms apply to short positions.
Profit or loss is realized when an open position is closed, meaning you sell to close a long position and you buy to close a short position. At that point, the PnL is given the term realized PnL. The total PnL at any point is the total of the realized PnLs so far and the unrealized PnLs for open positions at the market price. Here, we will discuss how trading ideas are born and how they are turned into algorithmic trading strategies.
Fundamentally, all trading ideas are driven by human intuition to a large extent. Intuitively, you may also reason that instruments that are very similar to one another, or loosely dependent on one another, will move together, which is the idea behind correlation-based trading or pairs trading. Since every market participant has their own view of the market, the final market prices are a reflection of the majority of market participants.
If your views are aligned with the majority of the market participants, then that particular strategy is profitable in that particular instance. Of course, no trading idea can be right all the time, and whether a strategy is profitable or not depends on how often the idea is correct versus how often it is not correct.
Historically, human traders implemented such rule-based trading to manually enter orders, take positions, and make profits or losses through the day.
Extracting data from the Quandl API
Over time, with advances in technology, they've moved from yelling in the pits to get orders executed with other pit traders, to calling up a broker and entering orders over the telephone, to having GUI applications that allow entering orders through point and click interfaces.
Such manual approaches have a lot of drawbacks — humans are slow to react to markets so they miss information or are slow to react to new information, they can't scale well or focus on multiple things at a time, humans are prone to making mistakes, they get distracted, and they feel a fear of losing money and a joy of making money. All of these drawbacks cause them to deviate from a planned trading strategy, severely limiting the profitability of the trading strategy. Computers are extremely good at rule-based repetitive tasks.
When designed and programmed correctly, they can execute instructions and algorithms extremely quickly, and can be scaled and deployed across a lot of instruments seamlessly. They are extremely fast at reacting to market data, and they don't get distracted or make mistakes unless they were programmed incorrectly, which is a software bug and not a drawback of computers themselves. They don't have emotions, so don't deviate from what they are programmed to do. All of these advantages make computerized automated trading systems extremely profitable when done right, which is where algorithmic trading starts.
Let's take a simple example of a trend-following strategy and see how that has evolved from a manual approach all the way to a fully automated algorithmic trading strategy. Historically, human traders are used to having simple charting applications that can be used to detect when trends are starting or continuing. This would be a classic manual trading strategy in the past.
As we discussed previously, computers are very good at following repetitive rule-based algorithms. Simpler rules are easier to program and require less development time, but computer software applications are only limited by the complexity that the software developer programming the computer can handle. At the end of this chapter, we will deal with a realistic trading strategy written in Python, but for now, we will continue to introduce all the ideas and concepts required prior to that. Here is some pseudo code that implements our trend-following, human intuition trading idea.
This can then be translated into whatever language of our choosing based on our application's needs. This variable tracks our current position in the market:. This is the expected profit threshold for our positions. If a position is more profitable than this threshold, we flatten the position and the unrealized profit to realized profit:. This is the maximum loss threshold for our positions. If a position is losing more than this threshold, we flatten the position and convert the unrealized loss to realized loss.
Why would we close a position if it's losing money? The idea is simply to not lose all of our money on one bad position, but rather cut our losses early so that we have capital to continue trading. More on this when we dive into risk management practices in more detail. For now, we define a parameter that is the maximum allowed loss for a position in terms of the price change from the entry price for our position:. This is not always symmetrical, but we will address the distributions of winning and losing positions when we look into these trading strategies in greater detail later in this book.
We need to check whether our signal causes an entry and whether one of our open positions needs to be closed for PnL reasons:. This is our entry signal to go long, so we will send a buy order and update our position. Technically, we should not update our position until the exchange confirms that our order matched, but for the sake of simplicity in this first-pass pseudo code, we ignore that complexity and address it later:. This is our entry signal to go short, so we will send a sell order and update our position:.
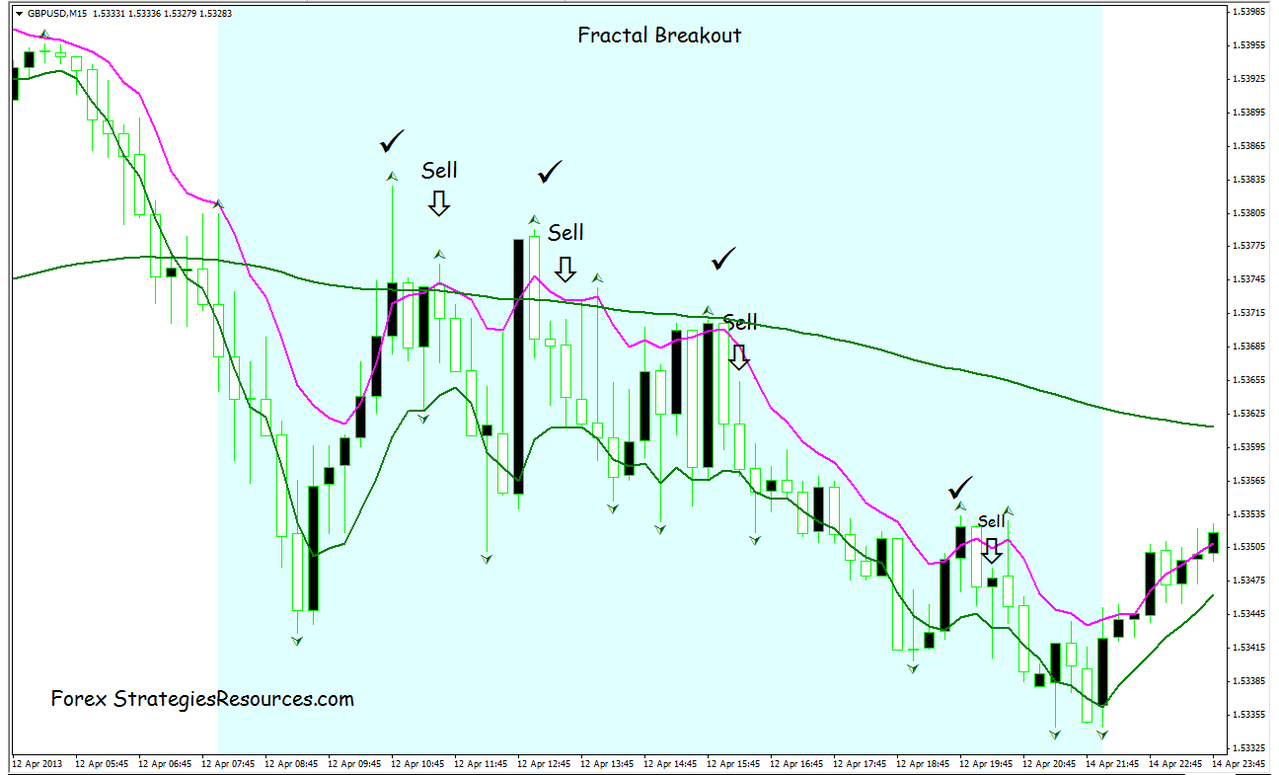
If we are currently long, and market prices have moved in a favorable direction, check whether this position's profitability exceeds predetermined thresholds. In that case, we will send a sell order to flatten our position and convert our unrealized profit to realized profit:. If we are currently long, and market prices have moved against us, check whether this position loss exceeds predetermined thresholds.
skills covered
In that case, we will send a sell order to flatten our position and convert our unrealized loss to realized loss. If we are currently short, and market prices have moved in a favorable direction, check whether this position profitability exceeds predetermined thresholds. In that case, we will send a buy order to flatten our position and convert our unrealized profit to realized profit:.
If we are currently short, and market prices have moved against us, check whether this position loss exceeds predetermined thresholds.
In that case, we will send a buy order to flatten our position and convert our unrealized loss to realized loss:. In an earlier section, we provided a top-level view of the entire algorithmic trading setup and many of the different components involved. In practice, a complete algorithmic trading setup is divided into two sections, as shown in the following diagram:.
These components are responsible for interacting with the feed handler components that publish normalized data.
- Python Programming Tutorials!
- Forex Trading using Python: Basics.
- lydia forex;
- Python for Finance – Algorithmic Trading Tutorial for Beginners?
- Forex Algorithmic Trading Strategies: My Experience | Toptal.
- Algorithmic Trading with Python | New Trader U.
This data can be delivered over a network or locally using a variety of Inter-Process Communication IPC mechanisms from the feed handlers. We do not go into great detail about this here. Low latency delivery and scalability are the major driving design decisions in this regard. Once the trading strategy gets normalized market data, it uses that data to build and maintain limit order books for each instrument. Depending on the sophistication and complexity of the limit order books, it can be simple enough such that it tells us how many participants there are on each side, or sophisticated enough to track market participant order priorities as well as track our own orders in the limit order book.
Once limit order books are built, every time they are updated due to new incoming market data information, we build signals using the new information. Signals are called by various names—signals, indicators, predictors, calculators, features, alpha, and so on — but they all have roughly the same meaning.
This is one of the areas where a lot of trading teams focus much of their time and energy. The key is to build a lot of signals in order to have an edge over the competition as well as keep adapting existing signals and adding new signals to deal with changing market conditions and market participants. We will revisit this in one of the later chapters, as this will be a large focus of this book. Often, a lot of algorithmic trading systems combine a lot of different kinds of signals in order to gain a bigger edge than individual signals provide. There are many different ways to combine individual signals.
You can use classical statistical learning methods to generate linear and non-linear combinations to output classification or regression output values that represent a combination of individual signals. Machine learning is not the focus of this book, so we avoid diving too deep into this topic, but we will revisit it briefly in a later section. Another key component of algorithmic trading is quickly and efficiently managing orders based on signals in order to gain an edge over the competition.
It is important to react to changing market data, changing signal values in a fast but intelligent manner. Oftentimes, speed and sophistication are two competing goals, and good execution logic will try to balance the two objectives in an optimal manner. Remember that other market competitors can observe what orders are sent to the exchange and assess the potential impact it might have, so this component needs to be intelligent enough to not make it obvious what our trading strategy is doing. Slippage and fees are also very important factors as far as execution logic design is concerned.
Slippage is defined as the difference in the expected price of a trade and the price at which the trade is actually executed. This can happen for predominantly two reasons:.
The Top 22 Python Trading Tools for 2021
Slippage obviously causes losses that might not have been correctly factored in, in addition to difficulty liquidating positions. As the position sizes for trading algorithms scale up, slippage becomes a larger problem. Fees are another issue with executing orders efficiently.
Typically, there are exchange fees and broker fees proportional to the size of the orders and the total volume traded. Again, as the position sizes for trading algorithms scale up, trading volumes typically increase and fees increase along with it. Oftentimes, a good trading strategy can end up being non-profitable because it trades too much and accumulates a lot of trading fees.
Again, a good execution logic seeks to minimize the fees paid. All algorithmic trading strategies need to track and manage their positions and PnLs effectively. Depending on the actual trading strategy, this can often range in complexity.